StreamTeam: From Individual Sensing to Collaborative Action Analysis (Finished)
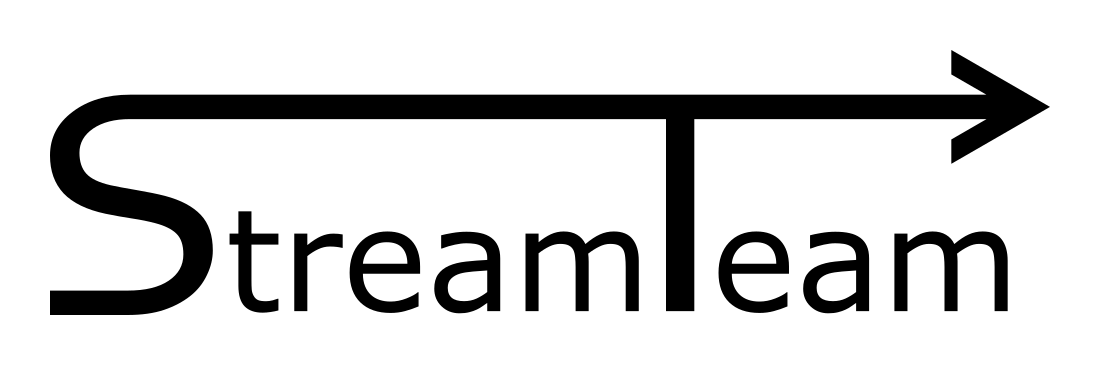
With the recent proliferation of small, unobtrusive embedded sensors, the number of data streams and the volume of streamed data has increased enormously. This has strongly influenced both our business and our private life and has brought forward a large variety of monitoring applications in different domains. In all these applications, the analysis of data streams in real-time is essential. One of the main challenges in data stream analysis is the detection of complex events out of the raw streaming data. While personal assistants allow to analyze in detail the activities of individuals, the analysis of entire teams, in particular complex events reflecting the interaction and relations between team members on the basis of the data streams captured on the individuals is largely unexplored. At the same time, such team analysis is essential in all applications where groups of individuals have to jointly solve complex tasks. Examples can be found, for instance, in team sports or in rescue operations (fire fighting, disaster management, etc.).
The goal of StreamTeam is to monitor, analyze, and visualize the actions of teams of individuals in real-time in highly dynamic and mobile environments in a robust and scalable way. StreamTeam meets the challenges arising from the very rapidly increasing volumes of continuous data streams that will be digitally captured on the individual and goes beyond mere data collection about sensed facts and log-based post-hoc analysis of the actions of individuals. Most importantly, StreamTeam takes a major step towards online inference of group behavior.
So far, StreamTeam provides i.) novel algorithms for the detection of semantic events in streams of data coming from highly mobile sensors, ii.) a scalable platform for the management of data streams and the detection of semantic events in real-time, and iii.) a modular approach towards specifying analyses (incl. complex events and high-level strategies) thereby bridging the gap between a generic platform and selected analyses in a concrete application.
As a next step, StreamTeam will be extended towards supporting more sports disciplines. Moreover, we will integrate deep learning approaches to detect complex events for which an algorithmic specification is not available. For this purpose, we will investigate if deep neural networks can be trained on the basis of existing (annotated) data sets. In addition, we will investigate if coach-specific data sets that are deduced from explicit or implicit event annotations in SportSense, our team sport video scene retrieval system, can be used to train coach-specific event detection networks.
The StreamTeam project will continue focussing on team sports, and in particular on football, based on the existing collaboration between the DBIS group at UNIBAS and the BFH Centre for Technologies in Sports and Medicine. As the Swiss Federal Institute of Sport Magglingen (SFISM) is part of the BFH centre, the latter acts as interface between technology and especially computer science and sports. The BFH centre closely collaborates with the Swiss Football Association (SFV) and in particular with the coaches of the youth national teams of the SFV. In addition, the BFH centre has also a close collaboration with the International Ice Hockey Federation (IIHF). As this community is currently in the process of preparing the basis for deploying sensors in the equipment of the individual players, ice hockey will be considered as a second use case for the evaluation of the project results as soon as these technical developments further proceed.
A recent video illustrates the collaboration between the DBIS group and the BFH Centre for Technologies in Sports and Medicine / the Swiss Federal Insitute of Sports (SFISM):
A video showing the real-time user interface of StreamTeam is published on Youtube:
In December 2017, an article on StreamTeam was published in Tageswoche.
Moreover, the whole code of StreamTeam is published on GitHub under the GNU Affero General Public License v3.0.
Start / End Dates
01.08.2015 - 01.01.1970
Partners
BFH Centre for Technologies in Sports and Medicine (Martin Rumo)
Funding Agencies
Funded since September 2017 by the Hasler Foundation in the Cyber-Human Sytems program (contract no. 16074).
Staff
Research Topics
Publications
2021
- Philipp Seidenschwarz
Data-Driven Analytics for Decision Making in Game Sports
PhD Thesis, Department of Mathematics and Computer Science, University of Basel, Switzerland 2021/9
2020
- Lukas Probst, Heiko Schuldt, Philipp Seidenschwarz, Martin Rumo
StreamTeam-Football: Analyzing Football Matches in Real-Time on the Basis of Position Streams
Proceedings of the IEEE International Conference on Big Data (BigData 2020) , Atlanta, GA, USA (held virtually) 2020/12 - Lukas Probst
Spatio-Temporal Multi Data Stream Analysis with Applications in Team Sports
PhD Thesis, Department of Mathematics and Computer Science, University of Basel 2020/7
2019
- Philipp Seidenschwarz, Martin Rumo, Lukas Probst, and Heiko Schuldt
A Flexible Approach to Football Analytics: Assessment, Modeling and Implementation
Proceedings of the 12th International Symposium on Computer Science in Sport (IACSS 2019), Moscow, Russia 2019/11 - Philipp Seidenschwarz, Martin Rumo, Lukas Probst, and Heiko Schuldt
A Flexible Approach to Football Analytics: Assessment, Modeling and Implementation (Abstract)
Book of Abstracts of the 12th International Symposium on Computer Science in Sport, Moscow, Russia 2019/7
2018
- Lukas Probst, Fabian Rauschenbach, Heiko Schuldt, Philipp Seidenschwarz, Martin Rumo
Integrated Real-Time Data Stream Analysis and Sketch-Based Video Retrieval in Team Sports
Proceedings of the 2018 IEEE International Conference on Big Data (BigData 2018), Seattle, WA, USA 2018/12
2017
- Lukas Probst, Frederik Brix, Heiko Schuldt, Martin Rumo
Demo: Real-Time Football Analysis with StreamTeam
Proceedings of the 11th International Conference on Distributed and Event-based Systems (DEBS 2017), Barcelona, Spain 2017/6
2016
- Lukas Probst, Ivan Giangreco, Heiko Schuldt
Pull-based Real-Time Complex Event Detection in Multiple Data Streams - the PAN Approach
Technical Report CS-2016-003, Department of Mathematics and Computer Science, University of Basel, 2016/8 - Lukas Probst, Ivan Giangreco, Heiko Schuldt
PAN – Distributed Real-Time Complex Event Detection in Multiple Data Streams
16th IFIP International Conference on Distributed Applications and Interoperable Systems (DAIS 2016), Heraklion, Crete, Greece 2016/6